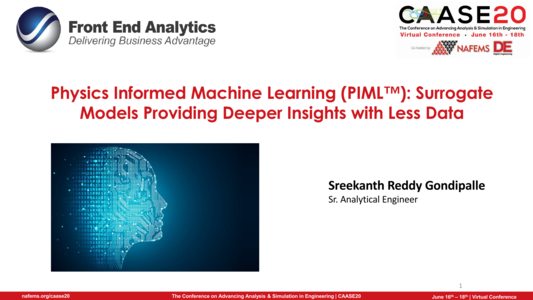
NAFEMS Americas and Digital Engineering (DE) teamed up (once again) to present CAASE, the (now Virtual) Conference on Advancing Analysis & Simulation in Engineering, on June 16-18, 2020!
CAASE20 brought together the leading visionaries, developers, and practitioners of CAE-related technologies in an open forum, unlike any other, to share experiences, discuss relevant trends, discover common themes, and explore future issues, including:
-What is the future for engineering analysis and simulation?
-Where will it lead us in the next decade?
-How can designers and engineers realize its full potential?
What are the business, technological, and human enablers that will take past successful developments to new levels in the next ten years?
Resource AbstractHistorically in the field of engineering, computational simulations such as Structural FE (Finite Element) Analysis, CFD (Computational Fluid Dynamics) analysis were conducted to predict the behavior of a design subjected to a specified set of boundary conditions. However, Simulations of complex real-world problems using Computer Aided Engineering (CAE) software tools may take hours or even days to compute. Due to this limitation, quite often these computationally expensive CAE simulations are not directly employed for design space exploration and optimization. However, Global product competition has placed greater importance for reduced product development cycles and for digital twin applications across several engineering fields.
Therefore, building efficient surrogate models or Reduced order models which are simplified mathematical representations of the complex CAE simulations, to predict the outcomes of complex engineering problems has become the need of the hour. Currently several data driven approaches such as Neural Networks, Radial Basis Functions, Kriging etc. are implemented to build these models. In order to build a good quality surrogate model, a sufficiently large training data set is typically created using Design of Experiments (DoE) strategy which will spread the designs uniformly across the design space. However, for expensive CAE problems; that may take days to compute, and have non-linear responses, building such DOE’s would take several months of computational effort if not more and so is not a feasible option.
So, we need to identify approaches to build surrogate models with smaller sets of training data; without compromising on the quality of the model built. Using physics informed machine learning (PIMLᵀᴹ) techniques developed at Front End Analytics, surrogate models are built over significantly smaller training data sets with a high predictive accuracy. This presentation provides an illustrative powertrain example that compares the development of a Surrogate Model using state-of-the-art machine learning method against PIMLᵀᴹ. The data obtained for the Surrogate Model was obtained from a Finite Element Modeling and Simulation.