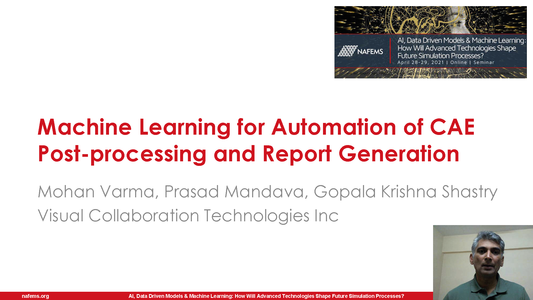
Artificial Intelligence (AI) is becoming more and more a part of the activities traditionally covered by the engineering analysis and simulation community. Recent advances in the application of AI, machine learning (deep learning) and predictive analytics, have brought these technologies to the fore in every area of industry.
This seminar hosted by the NAFEMS Americas Steering Committee brought together speakers from the end-user, consultancy, and academic industries to discuss where we are and how these technologies are being used to advance significantly the engineering analysis and simulation capabilities and approaches over the next 10 years.
Resource AbstractIn the product manufacturing industry, the significance of engineering simulations in the design and development of new products and product improvements has increased many fold over the past two decades. A typical engineering simulation workflow involves the CAE analysts pre-process and prepare models, run solvers to create simulations and finally post-process the simulation results and then document the insights and findings into CAE reports. These simulation insight reports play a major role in engineering decisions and design updates and are shared with the managers, designers & release engineers.
Rapid advances in computing hardware and processing power increased the size and complexity of the problems that are being simulated and reduced the turnaround time that the solvers take to provide the simulation results. The improvements in meshing algorithms lead to more tolerance for dirty geometries, increased the automation and reduced the manual effort and time required to be spent by the engineers and analysts in the preprocessing step. However, post-processing of the simulation results to identify the simulation insights and documenting them into actionable reports still remained as a predominantly manual process. A typical analyst spends a considerable proportion of his effort and time in manual post-processing and documentation. This process is very in efficient and it involves a significant amount of repetitive usage patterns. The lack of mechanisms to identify the repetitive usage patterns and to create robust and repeatable processes to post-process the engineering simulation results and to automatically extract the high fidelity actionable simulation insights into Digital CAE Reports is hampering the manufacturing industry to realize the engineering simulations’ full potential.
We at, VCollab, have been working to create solutions that allow users to create repeatable and machine learnable processes for processing the simulation results and automatic generation of “3D Digital CAE Reports” with high fidelity insights. The software system that we are working on would come with a library of predefined set of templates and reports. When a new model/solution is introduced into its database, it first extracts critical model metadata and identifies suitable report templates from its template database, based on the user history and the model metadata. These templates will be used to generate high fidelity 3D “Digital CAE Reports” containing 3-D views, 2-D plots and all the required annotations. Analysts can visualize the generated reports, edit them, do further post-processing in the browser, add additional content and save the updated high fidelity “3D “Digital CAE Report” back to its database. It avoids the pain of starting the post-processing from scratch for every new model/simulation and makes use of captured repetitive usage patterns. Machine learning algorithms will be used to update the classification/clustering mechanism based on the differences in the simulation metadata and the user changes to the reports, to identify suitable report templates that would be better for future model/solution files. This presentation provides additional details about the steps along with the use cases and demonstrations.