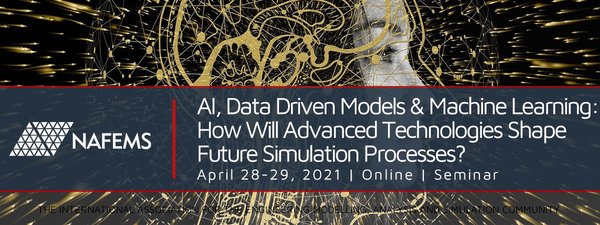
Artificial Intelligence (AI) is becoming more and more a part of the activities traditionally covered by the engineering analysis and simulation community. Recent advances in the application of AI, machine learning (deep learning) and predictive analytics, have brought these technologies to the fore in every area of industry.
This seminar hosted by the NAFEMS Americas Steering Committee brought together speakers from the end-user, consultancy, and academic industries to discuss where we are and how these technologies are being used to advance significantly the engineering analysis and simulation capabilities and approaches over the next 10 years.
Resource AbstractMachine learning and Reduced Order Modelling (ROM) techniques are interpolation methods exploiting data sets derived from existing virtual or experimental setup. They are essential to the concept of Digital Twins since they provide the missing link for both rapid re-design and operational evaluations in real-time. While the starting point is a DOE-type design with sufficient space filling properties, ML/ROM are different from response surface methods (RSM) where the approximation functions (surrogate model), often representing variations of a scalar entity within the design space, are imposed by the shape and nature of the fitting surface or a prescribed equation which also affects the number of runs required. ML/ROM techniques exploit the known physical behavior represented by the “modal” contents or similitudes expressed in terms of clusters of responses (ROM) in conjunction with various simple model prediction techniques (ML).
In some cases, ML/ROM can be considered as algebraic solutions, exploiting decomposition techniques of special and temporal components, to reduce the volume of data set while preserving the most important parts of the information contained within that data set, which is necessary for retrieving all or the most essential part of the information when needed. Otherwise, we can also consider ML/ROM as implementation of clustering or other “lossy” data compression techniques, allowing for the reduction of the amount of data required for the reconstruction of the complete data set. Both such techniques allow for creating on-board and real-time applications based on voluminous experimental or simulation results (ex. Finite element).
In this presentation, we shall provide state-of-the art robust and industrial solutions to typical applications in Crash & Safety, CFD, multi-physics and optimization. All have employed in one way or another.